Delivering Value at Scale
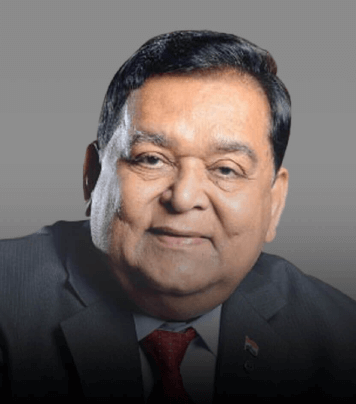
LTIMindtree is integral to L&T’s technology-led growth vision and is poised to play a crucial role in the expansion and diversification of our services portfolio. The highly complementary strengths of LTI and Mindtree make this integration a win-win proposition for all our stakeholders — clients, partners, investors, shareholders, employees, and communities — furthering L&T’s tradition of innovation, excellence, trust, and empathy.
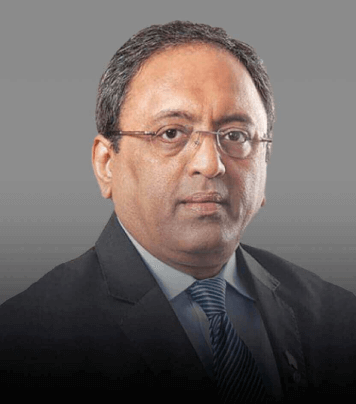
We are grateful to the authorities for the swift passage of the proposed transaction through statutory processes and for its approval in a record time. This integration is much more than just the coming together of two highly successful companies. It is about turning the collective wisdom of the two companies into a much larger force for creating long-term value for all our stakeholders.
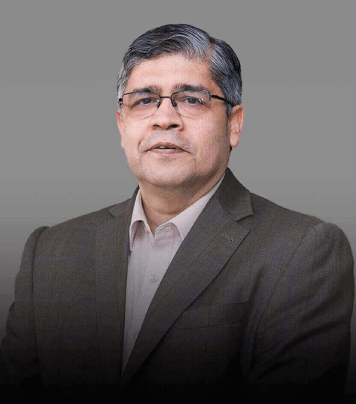
Amid the proliferation of new business models and revenue streams in a rapidly converging world, LTIMindtree will help businesses proactively take on and shape the future by harnessing the full power of digital technologies. Armed with top talent, comprehensive offerings, and a cumulative experience of more than five decades, LTIMindtree brings the diversity of scale and capabilities required to help businesses reimagine possibilities, deliver impact, and get to the future, faster.
Creating Next Level Growth
Revenue
Clients
Employees
Countries
Unmatched Core to Experience Transformation
LTIMindtree is a new kind of technology consulting company. We help businesses transform – from core to experience – to thrive in the marketplace of the future. With a unique blend of the engineering DNA with the experience DNA, LTIMindtree helps businesses get to the future, faster.
Together Creating Value
Partners
Client Speak
LTIMindtree played a pivotal role in Gen Re's cloud migration journey, addressing scale challenges and geographic diversity with a highly skilled team. Their comprehensive approach ensured a seamless transition, meeting Gen Re's objectives successfully. Erik Kasir, VP at Gen Re, commended LTIMindtree for their strategic selection, effective risk mitigation, and collaborative teamwork.
Johan Gabrielsson, Head of Digital Products & Services at OKQ8, talks about their long-lasting partnership with LTIMindtree in Digital Products & Platforms. The association has expanded from early IT development to creating innovative apps for sustainable mobility. OKQ8 and LTIMindtree's partnership has now shifted towards a strategic emphasis on harnessing data to make substantial advancements in the digital arena.
Giving wings to one’s business in turbulent skies seems to be the mantra for the CIO of Lufthansa Cargo AG - Jochen Gottelmann. He speaks about how Lufthansa Cargo responded to the pandemic with agility and resilience, and how LTIMindtree helped drive it’s ESB middleware transformation. In Jochen’s words, LTIMindtree has proven to be a reliable, competent, scalable & trustworthy partner – both, in good times and in not so good times.
Providing the leading technology platform was not the only deliverable for LTIMindtree through MuleSoft. People and processes are equally important. That’s how, LTIMindtree successfully delivered this challenging, yet engaging and resourceful solution to Hoist Finance.
Success Stories
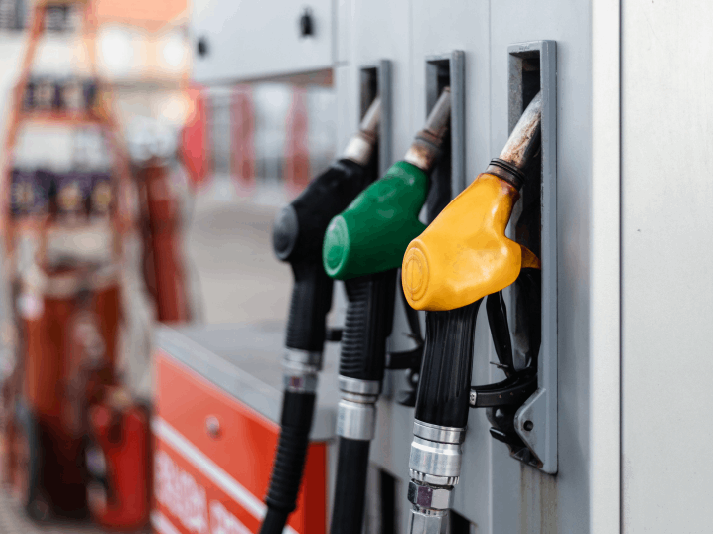
Driving Sustainability through Cloud Transformation for OKQ8
OKQ8's Azure journey exemplifies sustainability and innovation, setting new standards in responsible business practices.
Read More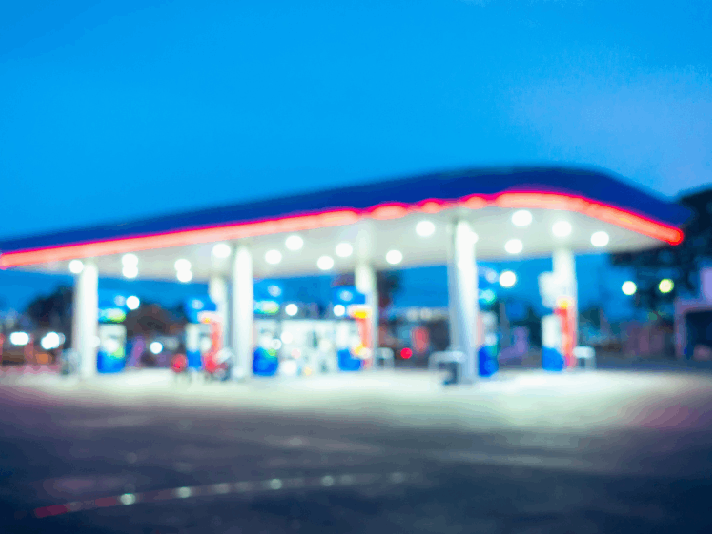
Cloud Cost Savings with Strategic Optimization for OKQ8
OKQ8 saved SEK 3 Mn with Azure's proactive monitoring, right-sizing, and resource alignment, ensuring efficiency and scalability.
Read More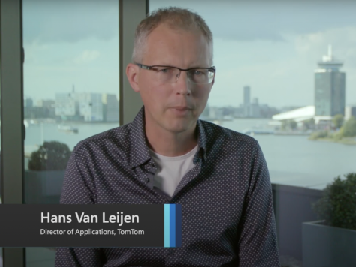
TomTom uses Microsoft Azure to modernize their applications
LTIMindtree helped TomTom scale & achieve high availability by migrating from on-premises to cloud and modernizing their applications with Microsoft Azure.
Watch VideoRecognitions
LTIMindtree has been awarded the prestigious nasscom Spotlight Award for Best-in-Class R&D Organization! The Spotlight Awards recognizes organizations that are making significant contributions to the Research & Development landscape.
Read More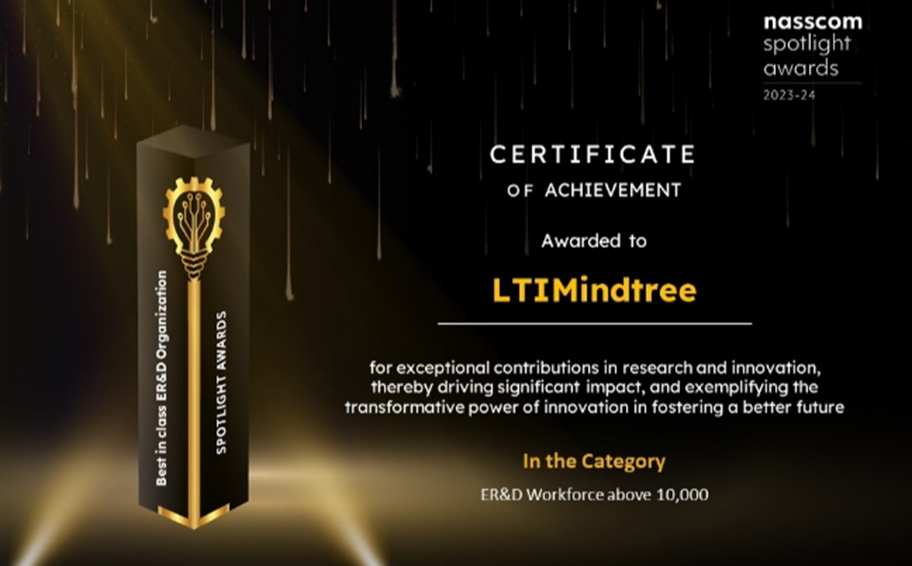
Recognitions
LTIMindtree has made it to the Carbon Disclosure Project (CDP) Global Leaderboard for the fourth consecutive year by scoring an "A-" in the 2023 Climate Change Ranking
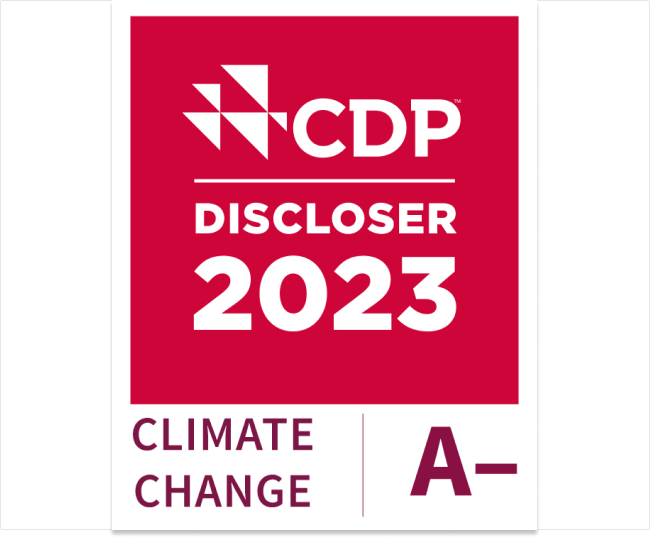
Recognitions
LTIMindtree Receives Top Honors for Sustainable Reporting Practices at the ICAI Sustainability Reporting Awards 2023
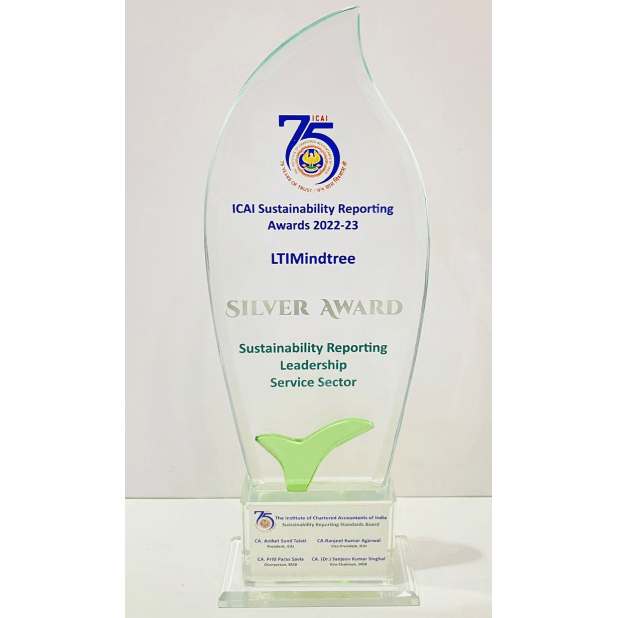
Recognitions
LTIMindtree has been awarded First Runner-Up for Disability Confidence & Inclusion, Second Runner-Up for LGBTQIA+ Inclusion, and First Runner-Up for DEI Champion at the Bombay Chambers DEI Awards.
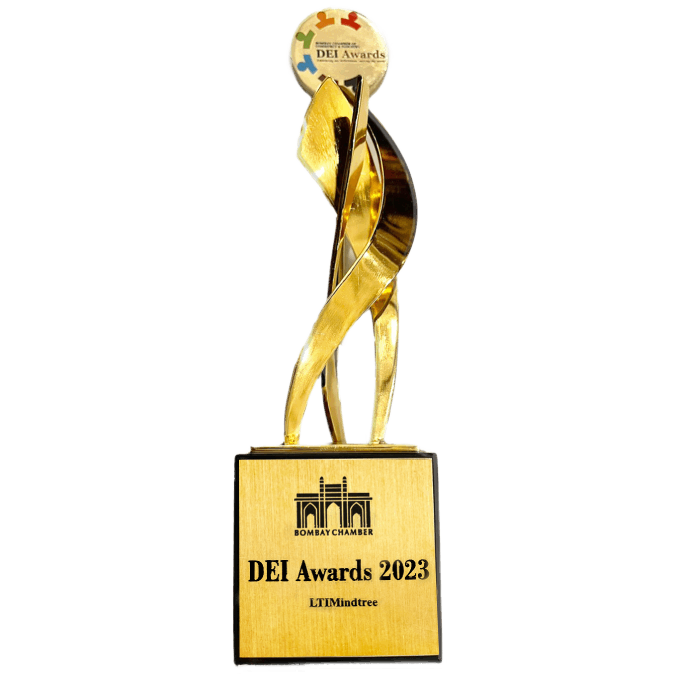
Recognitions
LTIMindtree Recognized as a Great Place to Work in Poland
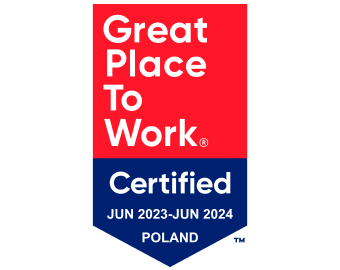
Recognitions
LTIMindtree honored at the 17th CII-ITC Sustainability Award 2022 as a prestigious winner for our commitment to excellence in Corporate Social Responsibility
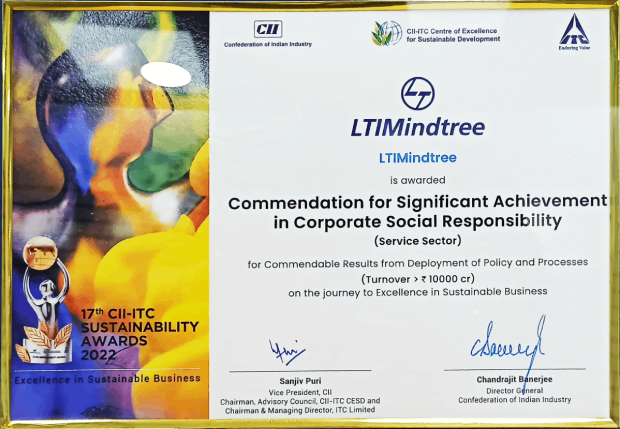
Recognitions
LTIMindtree’s GeoSpatial NxT honored for Excellence in Innovation at the IoT Evolution IoT Excellence Awards 2022
Read More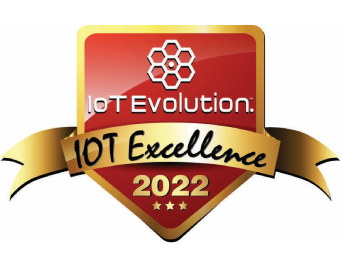
Recognitions
LTIMindtree Recognized as a Top Employer in the UK for Third Time in a Row
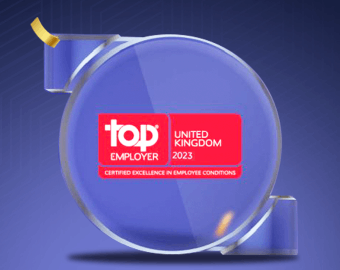
Recognitions
Debashis Chatterjee, LTIMindtree CEO and MD, Honoured as Transformational Leader for 2021 by Asian Centre for Corporate Governance & Sustainability
Read More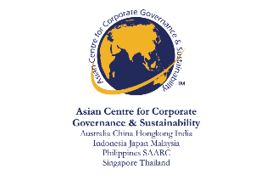
Recognitions
LTIMindtree has been featured in Ad Age's Datacenter Agency Report 2023, as one of the Top 25 Agency Companies. We are proud to be the only agency company based in India to make this list. As a major player in the business transformation field, we generated 65% of our global revenue from transformation services in 2022, amounting to USD 333 million.
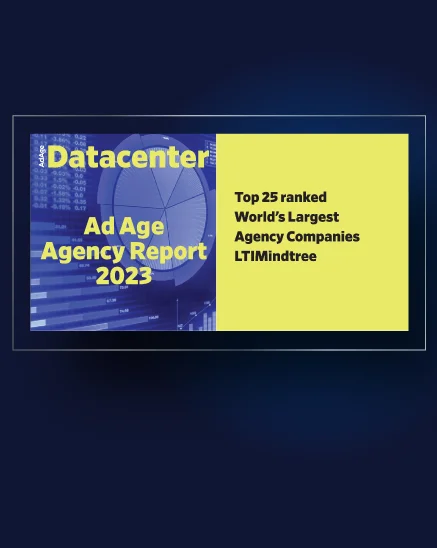